This is the final blogpost of the AI Research and COVID: Journeys to Gender Equality and Inclusion series. This blog series emerged from the “writeshop” organized by Gender at Work as part of the Data Science and Artificial Intelligence Research Program to Combat COVID-19, also known as AI4COVID, financed by the International Development Research Centre (IDRC) and Swedish International Development Cooperation Agency (SIDA). The initiative was part of the final Gender Action Learning workshop held in Nairobi, Kenya in February 2023, and is part of the greater context of Gender at Work’s partnership with IDRC to advance gender equality in development research.
In this blog post, Carol Miller and Marie-Katherine (Kate) Waller reflect on the key threads of this blog series that emerged from Gender at Work and research grantees’ collaboration in an 18-month gender action learning process in the AI4COVID program. They also highlight how researcher journeys, and the GAL process demonstrated the importance of creating safe spaces for learning and the value of multidisciplinary collaboration in supporting social transformation.
Our journey into gender equality and inclusion in AI research and COVID began in December 2020 at the height of COVID-19. It was the first AI4COVID program inception meeting for research grantees to meet each other, including Gender at Work, and to discuss responsible AI and gender equality and inclusion (GEI) aspects in grantee research.
Together with our International Development Research Centre (IDRC) AI4COVID program officers, we listened and heard: In responsible AI, what is wrong or right and at what stage? What are the principles and practices? Can we have minimal benchmarks of ethical and fair AI? In gender equality and inclusion, what data collection methods should we use? How can we ensure diversity of representation? How do we get this data?
From this moment onwards, we were hooked. As applied feminist research practitioners, we shared the same passion to ensure the most vulnerable and excluded groups are not left behind. Our task was to support research teams to enhance their GEI approach and related results. Our core approach was to facilitate an 18-month gender action learning (GAL) process. This involved tailored mentoring and support to each research team, by a Gender at Work (G@W) or Ladysmith facilitator, on gender equality and inclusion. This was combined with three peer-to-peer interactive workshops every six months in which research teams explored and learned together about ‘how’ and ‘what works’, in order to integrate gender and inclusion into AI-driven health research. The objective was to deepen understanding and collective approaches for more gender equitable research and outcomes.
This blog series, which emerged from the final GAL workshop and writing sessions organized by GAW and Ladysmith, in partnership with IDRC, captures the team journeys we witnessed. These stories reflect individual researchers’ personal and professional experiences of integrating a gender equality and inclusion lens into AI-driven health research.
Their stories speak to the complexity of gender, inclusivity and intersectionality in the world of AI and health. And while there are no simple answers to the question posed above, this final blog in the series draws together a few common threads. From these researchers’ journeys, we observed several patterns emerging from the fabric of their shared experiences.
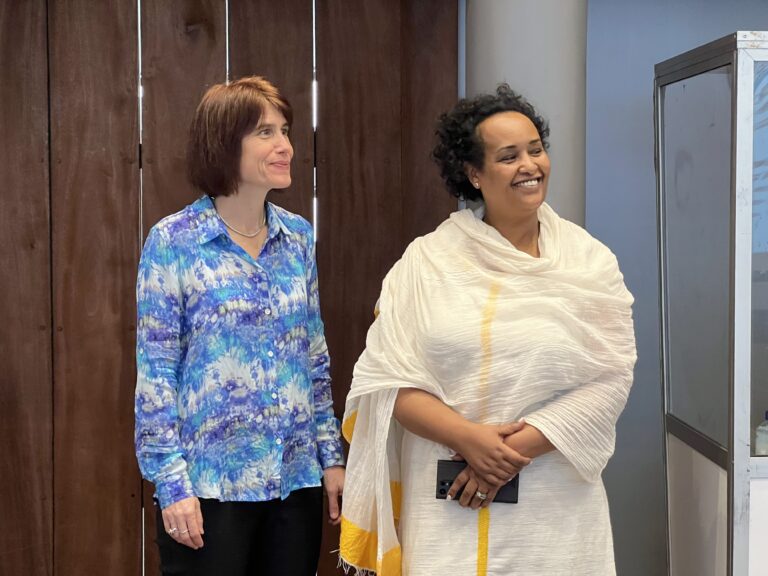
Taking a GEI lens starts with self-reflection
We learned that questioning our taken-for-granted ideas about gender-related norms is often the first step. Meghan Malaatjie’s story “Are women programmed to think less and do more?” and Sylvia Kiwuwa Muyingo’s “A Biostatistician’s Personal Journey through Gender Bias” ask us to consider how we were raised and to think about the roles of men and women in the African context — whether from the perspective of a young inspiring female academic, or through the eyes of a researcher conducting community field work and noticing women as primary caregivers to the chronically ill.
To understand gender inequalities in health requires both self awareness as researchers and collective awareness as research teams. This includes building the practice of constantly asking ’why’: for example, Michelle Mbuthia in “Cook, Clean, Plan: A case for more gender-responsive policymaking” wonders why women are expected to take on the heavy burden of all care work, which worsened in disease outbreaks. She challenges taken-for-granted gender norms and roles, and calls on both women and men from her family, and those leading her country, to question and change such unequal gender roles in favour of equal sharing of such responsibilities.
This is no easy task. It demands a willingness to listen, learn and unlearn.
Learning to listen to unlearn
From these blogs, we also learned about the importance of listening.
The act of writing the blogs motivated Jim Todd in “Break out of your silo” to share his experience of learning to listen, and hear a female colleague and woman of colour’s lived experience, regardless of being a white privileged male academic. He acknowledges that each of us lives in relationships of power and privilege. Depending on the context, we may be the one with more power, or the one with less.
In the world of global health and AI-driven health research, for example, there is increasing attention paid to the lack of women’s leadership, particularly of women from low and middle-income countries, and of female scientists and AI developers. In “Gender Marked Me: The Value of Gender Analysis in AI Research,” Tidiane Ndoye opens up about appreciating and respecting female role models, whether a mother or a female colleague, and how these role models can help foster happier and better men.
The experiences shared by the bloggers brought home to us that we need to get out of our own siloes ― or echo chambers ― and into potentially uncomfortable situations where our assumptions are challenged. Ethan Gilsdorf, in “No Differences, Only Sames: Finding Common Ground in Nairobi During the AI4COVID Gender Action Learning Writeshop,” illustrates how through travel we are put into situations where we realize, despite differences, we are the same. We can begin to confront others’ realities and unlearn some of our biases. Because these experiences are deeply human, differences of nationality, gender or socioeconomic backgrounds does not mean we cannot understand each others’ suffering or joy.
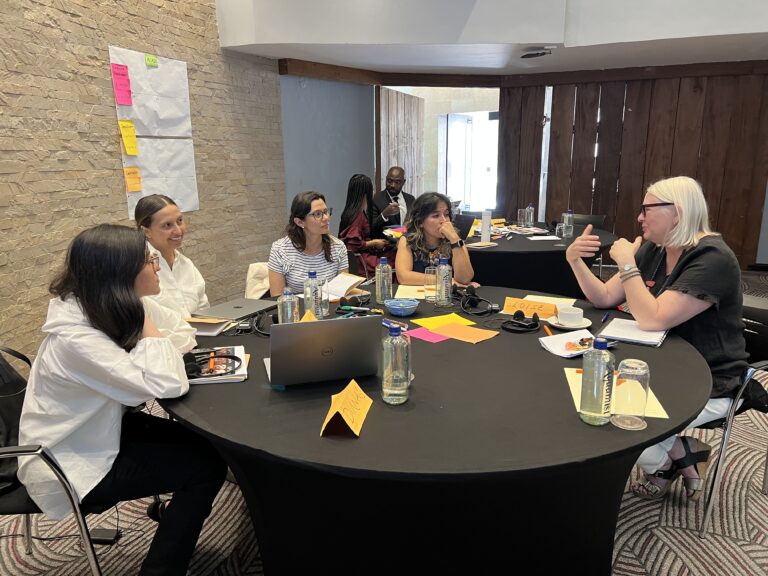
Debiasing data requires cultural and contextual sensitivity
We discovered that from an AI data scientist perspective, developing an algorithm requires tough decisions to be made around data collection and analysis. Biostatisticians, AI data scientists and social scientists spoke of the need for AI-driven researchers to question and contextualize data by cultural differences. Amelia Taylor, in “Can AI Have Its Cake and Eat It? Reducing Bias in AI Models May Not Always Be Desirable,” raises the complex and biased role of heuristics (problem-solving by experimental and trial-and-error methods) to arrive at algorithms.
In her blog “Gender and health data on the Venezuelan migrant population in Colombia,” Sandra Patricia Martínez-Cabezas shows how health records of Venezuelan migrants were heavily skewed towards women and children and neglected men’s health. We learned that data needs to be representative of the communities that it may impact to avoid excluding certain groups over others.
Intersectionality must be a criteria for data quality
We became aware that multidisciplinary AI-driven health research for combating COVID-19 and future pandemics must be grounded in gender and intersectional approaches. Hélène Agnes Diène’s “My adventures with COVID-19 under the lantern of intersectionality” discusses the importance of using heterogeneous datasets and gender and intersectional analysis in order to capture the multi-dimensional factors of vulnerability to infectious disease. Data, at a minimum, needs to be disaggregated by sex, gender, and age. The AI applications ― and ultimately the policies and programs that are generated from more intersectional nuanced data and analysis ― are critical to all levels of health and AI ecosystems in order to have fair, gender-just and effective AI solutions that leave no one behind.
Creating innovative, safe spaces for learning on gender is possible
For Gender at Work, the past two years working with the multidisciplinary research teams who were part of AI4COVID has also provided a fertile ground for our own growth about how to support innovative and safe spaces for learning on gender, equity and inclusion. We learned to adjust our trademark Gender Action Learning (GAL) processes, which had always been in-person, to the virtual world.
In “Why are you talking to a blank screen,” our G@W colleague Mahlet Hailemariam reflects on the importance of making every effort to build relationships of trust and collaboration, despite weak internet signals and feelings of exclusion because you cannot connect properly or no one can hear you.
From the in-person workshop in Nairobi, we realized that having people together from around the world is more powerful than our online GAL journey. However, we observed that the latter did help build the foundations for the former because people felt they already knew each other. We learned that online facilitation requires a delicate balance of building trust while creating opportunities for participants to share their research and to get right to the heart of the most critically reflective questions. We also learned the value of interactive online tools like jamboards and breakout rooms.
For our final GAL in-person workshop, we learned from our workshop evaluations that (1) a mixed method of learning how to use a participatory evaluation method, (2) outcome harvesting combined with storytelling, and (3) use of participatory methods like appreciative inquiry and world café were appreciated for enabling participants to understand both the types of changes their research projects have supported, and to take a deeper dive into understanding how these changes happened.
Another thread that emerged from our experiences and the blogs is that gender, exclusion, AI and public health issues are shared problems needing the conceptual and practical technical approaches of a broad range of pure and social scientists. This was part of the initial thinking behind the IDRC project. Still, as feminist researchers, our experiences engaging with the multidisciplinary teams really drove home the value of this approach. Just as the mix of perspectives and skills from different disciplines is important, so is the diversity in gender, sex, age, and backgrounds that these scientists and data experts bring to the research. This is the kind of research collaboration required to support social transformation.
Finally, we learned that despite our different nationalities and backgrounds, if we can acknowledge our own biases, we are then better able to understand how systems of discrimination shape our worlds, including COVID and AI ecosystems.
This blog post was written by Gender at Work Associates Marie-Katherine Waller and Carol Miller, & is licensed under a CC BY 4.0 license. © 2023 Marie-Katherine Waller and Carol Miller.
Curious to read the reflections on gender inequality, exclusions, & AI from this series? Read the other blog posts here.